Very long-period and ultra-long-period seismic signals detected before and during the formation of the Dolomieu caldera on Reunion Island in 2007
Caldera collapses (giant circular or elliptical volcanic craters formed by the emptying of the magma chamber) are rare phenomena. Only seven have occurred in the last hundred years. These events can bring about major, even catastrophic, changes to the landscape, the environment and the activity of volcanic edifices.
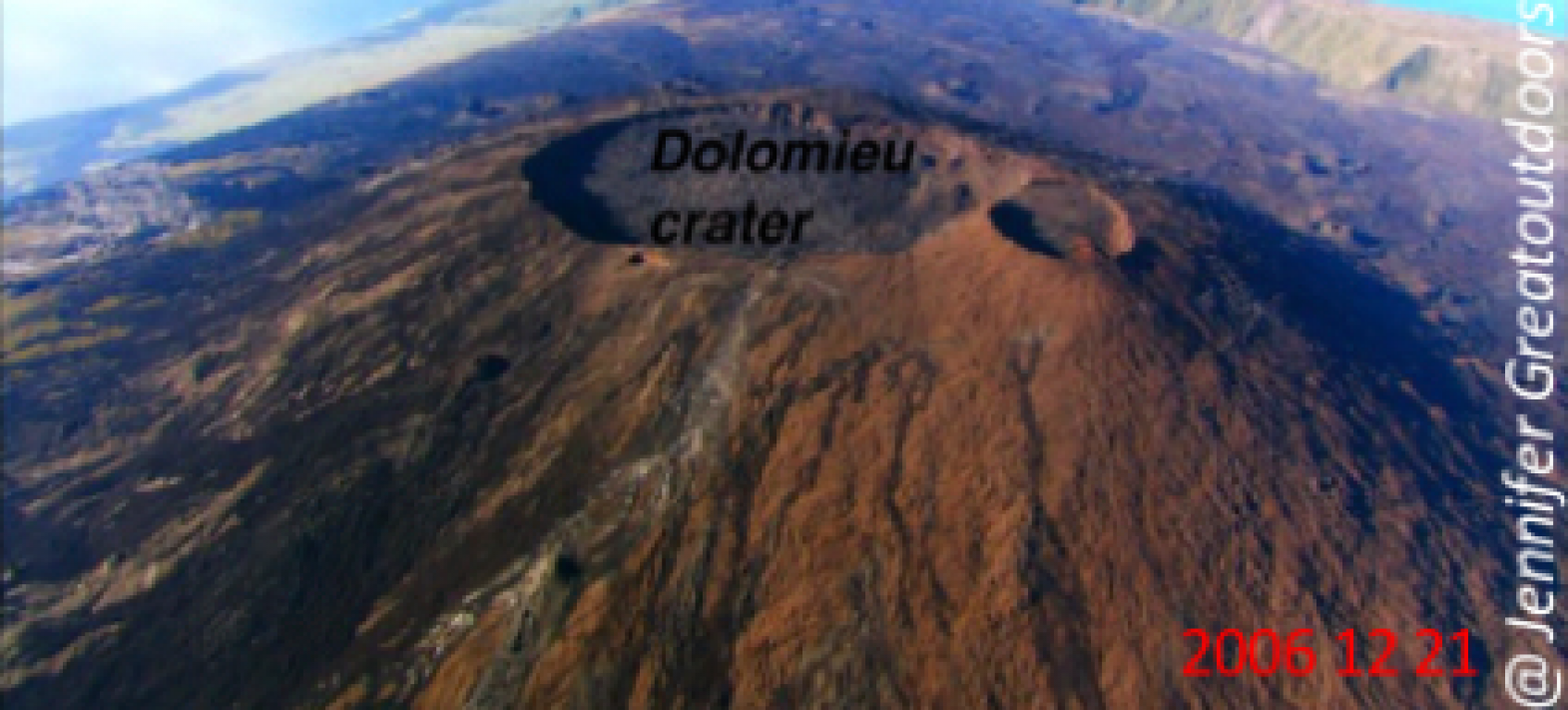
Publication date: 07/06/2019
Observatories, Press, Research
Related observatories : Volcanological Observatory of Piton de la Fournaise (OVPF-IPGP)
Related teams :
Volcanic Systems
Related themes : Natural Hazards