Analysis of InSight mission data using supervised and unsupervised Deep Learning algorithms
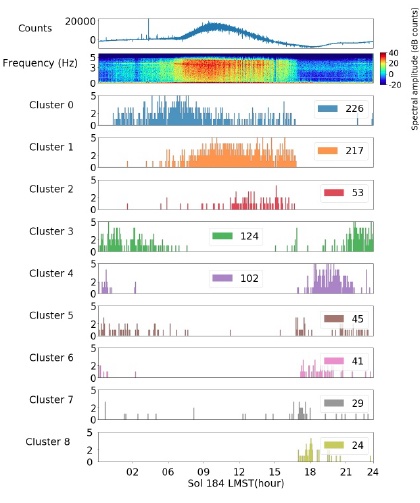
Start: 01 October 2017
End: 13 December 2021
Supervisors :
Philippe Lognonné
Related observatories :
InSight Observatory
Related teams :
Planetology and Space Sciences
Status: Defended
Planetary seismology has proved to be a powerful tool to decipher the interior structure of planets and to monitor their seismic activity. As illustrated by Insight (Interior Exploration using Seismic Investigations, Geodesy, and Heat Transport), which is operating the first geophysical observatory on Mars since February 2019, highly sensitive seismic measurements remain however challenging, due to both the limitation inherent to space exploration and the weak seismic activ-ity of terrestrial bodies other than Earth. For Mars in addition, we have an active environment generating a structured noise which might be difficult to understand with methodologies devel-oped by seismologists for the Earth.
This PhD dissertation is focusing on overcoming this problem by developing new tools based on Machine Learning and Deep Learning algorithms and explore both unsupervised and supervised Machine Learning approaches.
The two first chapters introduce the thesis. The first chapter is an overall introduction of plane-tary seismology with a focus on the InSight mission and its data. It provides also is a description of the state of the art of machine learning in seismology, in order to highlight their applications in planetary seismic data analysis as well as solving inverse problems in seismology to estimate the interior structure. The second chapter is a detailed description of the method of Machine learning, with focus on those used in this PhD on the InSight data.
In the third chapter, we discuss the seismic noise recorded by the InSight seismometer which is revealing numerous transient micro-events, associated mostly to an active Martian environment with wind bursts, pressure drops, in addition to lander and instrument thermally-induced cracks and vibrations. Identifying these micro-events is a mandatory first step prior the identification of marsquakes and the search of secondary phases. This has been made until now mostly by the operators of the In-Sight Mars Quake Service (MQS). Considering the low amount of labeled data from the SEIS instruments, and in general, the absence of labeling at the beginning of any new mission in a new environment, we investigate the continuous data with an unsupervised deep learning algorithm built on top of a deep scattering network. We show that our approach leads to the successful detection and clustering of these micro-events. We first provide a detailed description of our approach and present the learned clusters by investigating their origin and their physical properties. We show that the clustering is stable over several sols and allow us to identify sequences of glitches that repeat at a rate of several tens per sol with stable occurring time differences. We then show that the clustering and detection efficiency for pressure drops and glitches, some of the clusters found, is superior to manual or targeted detection techniques proposed to date. We finally discuss the impact of the noise structure on some of the already published works and conclude on the risk to over interpret either secondary phases on raw seis-mograms or phases in auto-correlation analysis.
The forth chapter of the Ph’D presents a new methodology to detect the Martian seismic events automatically with a supervised bidirectional long-short term memory network built on the deep scattering network for automatic features extraction. This approach is based on the learning of the MQS catalog and try to reproduce it, in order to make a new automatic tool to detect marsquakes including the events start. We present in detail the first results and show that such an approach can detect the majority of the events with good signal to noise ratio and more than half of those with a bad signal to noise ratio. The implementation of this method in the InSight ground operations is therefore an important perspective for the near future.
. We conclude by discussing the middle term perspective, not only for the InSight mission, for which we can not only envisage the application of an automated detection, based on the finding of the chapter 4, but also interesting perspectives in terms of prediction of the atmospheric noise in the seismic data. These machine learning methods can also be considered for Earth or futur Space geophysical stations, especially when the transmission of data is difficult and severe data selection must be made onboard.