Spatio-temporal study of seismicity in subduction zones from inhomogeneous seismic networks with artificial intelligence
Better understanding of the dynamics of large subduction earthquakes with machine learning
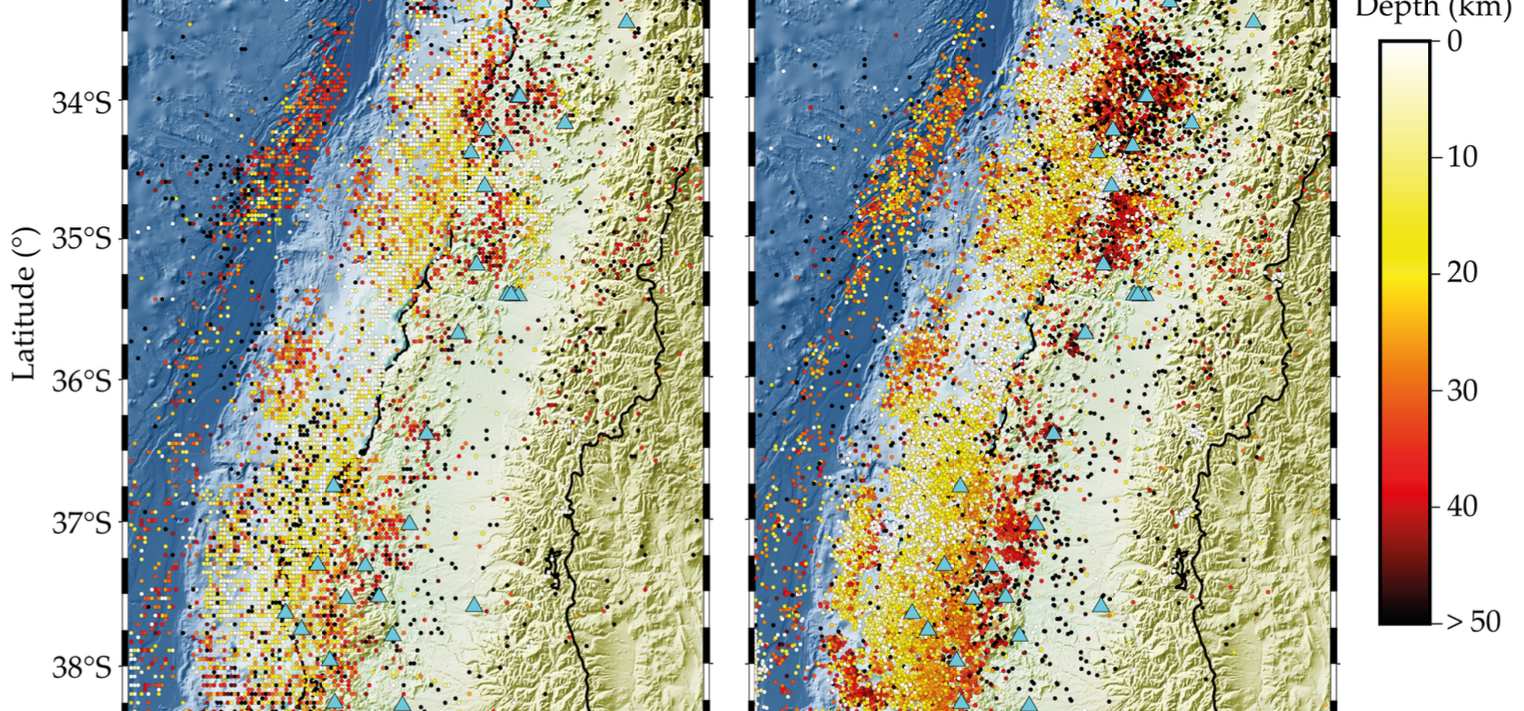
Start: 02 October 2023
End: 30 September 2026
Supervisors :
Léonard Seydoux, Fabian Bonilla
Related teams :
Seismology
Status: In progress
Understanding the mechanisms that govern earthquakes and post-seismic deformation is essential for evaluating natural hazards and devising efficient mitigation strategies. Over the past two decades, the availability of continuous seismic records have illuminated various types of earthquakes, characterized by varying durations and magnitudes. These phenomena underscore the vast range of physical processes at work within subduction zones at the interface between tectonic plates. Gaining a deeper understanding of these processes is crucial for constraining the occurrence of large, devastating megathrust earthquakes. In addition, these earthquakes often produce intense ground motion, thereby weakening the Earth’s near-surface materials and sometimes causing liquefaction. Analyzing the seismic response, which entails identifying the medium’s resonant frequencies and amplification, enables scientists to closely monitor the behavior of shallow-crust materials. In particular, unconsolidated sediments are prone to frequency shifts and velocity changes during strong motion. Investigating these sediments can yield valuable insights into nonlinear effects and temporal changes in the upper crust, ultimately contributing to understanding the dynamics at play during seismic events.
Nowadays, digital seismograms are continuously collected in seismogenic zones to monitor various parameters such as deformation, seismicity migration, and nonlinear effects. However, spatially dense continuous records have only been available for one or two decades, thus providing a short-term observational window (e.g., decades) when compared to the full seismic cycle (e.g., several decades to centuries). The distribution of seismic sensors remains notably inconsistent in space and time, resulting in incomplete seismic catalogs for certain regions. While the detection and location of larger earthquakes are generally straightforward due to high signal-to-noise ratio, weaker local seismicity proves more challenging to be accurately identified because of scattering and attenuation. Many existing earthquake catalogs are incomplete, particularly in regions with sparsely deployed seismic stations. This limitation motivates the need for enhanced strategies to obtain more comprehensive data on seismic activity and the associated phenomena, ultimately leading to improved understanding and mitigation of seismic hazards.
Advanced techniques such as template matching have significantly improved our capacity to detect low-amplitude seismicity. Template matching involves comparing pre-identified earthquake records with continuous data to identify similar events. The efficacy of template matching strategies depends on three key assumptions: (1) it requires the identification of seismicity throughout the entire area of interest, which is typically achieved using conventional detection techniques, (2) it presumes that the wave propagation medium remains stable enough for events with similar mechanisms occurring within a given area to exhibit comparable waveforms, and (3) the success of template matching relies on the continuous availability of array seismic data, which may not always be feasible due to factors such as maintenance costs, terrain constraints, or both. Moreover, dense seismic arrays are often deployed in regions where a large rupture has taken place, as it may be impractical to continuously monitor vast areas. This constraint significantly prevents us from forecasting seismicity during inter-seismic periods. To overcome these limitations, further advancements in detection techniques and strategies are necessary for enhancing our understanding of seismic activity and improving our ability to mitigate associated risks.
In this PhD proposal, our objective is to devise innovative strategies that improve the template matching method, enabling more accurate detection and location of weak seismicity during aftershock sequences following large earthquakes, specifically within subduction areas such as along Central and South America, Cascadia, and Japan. Additionally, we will address the challenge of enhancing the detection capabilities of sparse seismic arrays originating from dense deployments, which were established in response to major ruptures. Our goal is to observe the interseismic period and identify any potential pre-existing seismicity migrations prior to the main shock, including the detection of slow earthquakes such as low-frequency earthquakes and tectonic tremors.
The a priori detection of seismic templates is a critical step in enhancing the detection of similar events and subsequently enriching the catalog of seismicity. Given a stable seismic station deployment over a specific time period, we will employ cutting-edge AI-based methods to detect earthquakes within continuous seismograms. In particular, we will explore PhaseNet, a deep neural network designed to identify P and S seismic phases from seismograms, along with other similar strategies. Using this information, we will locate the earthquakes with an a priori velocity model obtained for the area and construct a template-matching catalog with a lower completeness magnitude than existing ones. This segment of the PhD research will start on the aftershock sequence of the 2010 Mw8.8 Maule earthquake in Central Chile. Over 40 broadband accelerometers and velocimeters were deployed following the mainshock, yielding dense, continuous seismic data for one year after the rupture. We will develop and apply our method to this case before conducting comparative studies in other subduction zones, gradually expanding to other regions in South America towards Mexico and beyond in Cascadia. The second portion of the PhD research will involve analyzing the migration of seismicity and comparing it with external observations, such as GNSS time series. The objective of this part is to investigate the post-seismic deformation following the mainshock and examine the nonlinear effect of the crust through ground motion analysis. After investigating these two stages, we plan to utilize AI techniques to transfer the knowledge acquired from a specific subduction zone during the dense deployment to past data with sparser spatial coverage of instruments, searching for past weak seismicity in the time period preceding the mainshock. This strategy can then be applied to other subduction contexts, such as Mexico and the Cascades, further advancing our understanding of seismic activity and enhancing our ability to predict and mitigate seismic risks.
To address these questions, we will collaborate with international partners from the United States, Chile, and France. We will work with William Frank from MIT, on template matching, Sergio Ruíz from Universidad de Chile to study seismic migration, and Philippe Gueguen, ISTerre, to investigate nonlinear seismic response.
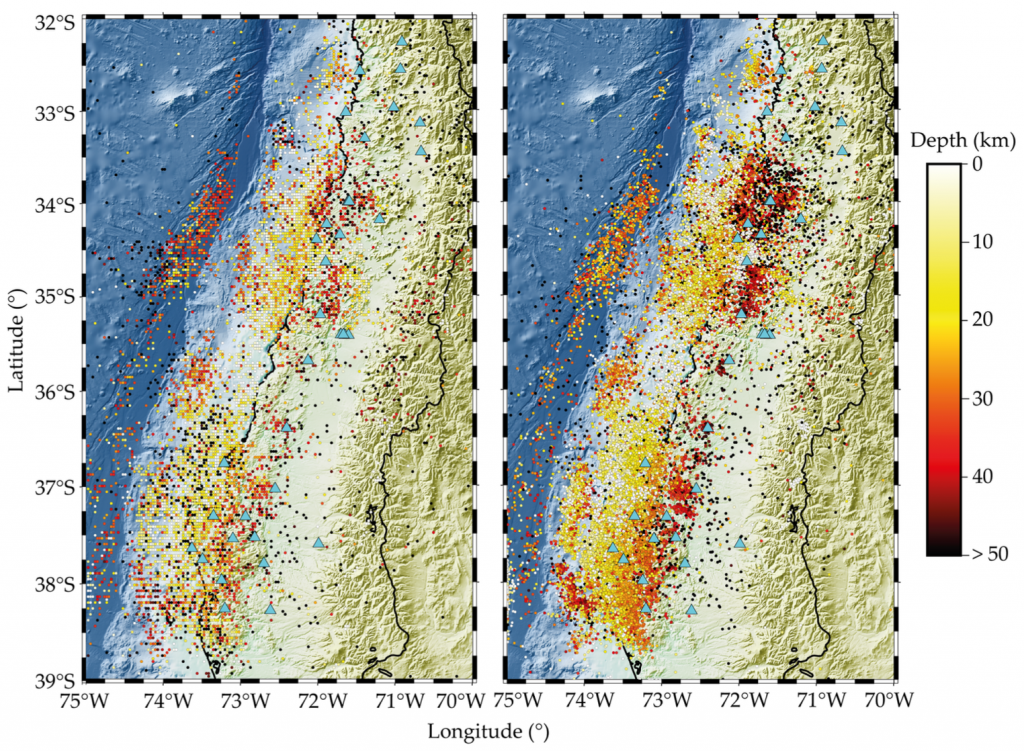
Illustration comparing the preliminary earthquake locations and enhanced locations within the Maule earthquake rupture zone. Each map depicts the available seismic stations, marked in cyan, spread across the study area. The color bar on the right represents the depth of the earthquakes, up to a limit of 50 km. Crustal and shallower events are colored in yellow and almost white, while deeper earthquakes related to megathrust subduction activity range from red to black.