AI-based seismovolcanic activity monitoring of dormant volcanic systems
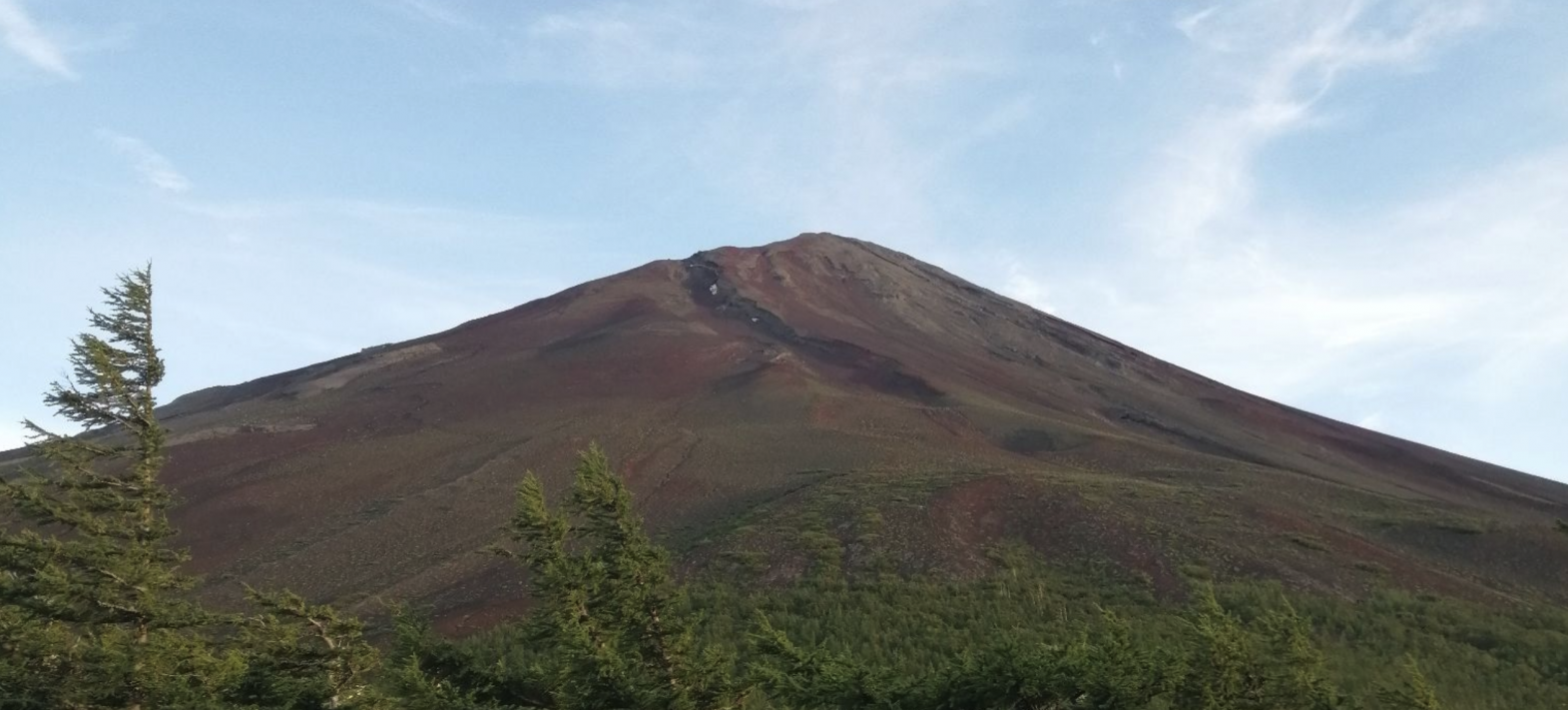
Start: 02 October 2023
End: 30 September 2026
Supervisors :
Jean-Philippe Metaxian, Léonard Seydoux, Nobuaki Fuji
Related teams :
Sismologie, Seismology
Status: In progress
Mount Fuji has been inactive for more than three centuries. Yet, an eruption could potentially impact 30 million people. Despite its apparent dormancy, Mount Fuji is seismically active and tends to produce an increasing number of volcanic low-frequency earthquakes over time. This PhD proposal aims to use seismic imaging technique and artificial intelligence to detect, locate and characterize the seismic activity and structural changes of Mount Fuji and better constrain the volcanic risk in its vicinity. Our project seeks to provide an efficient workflow for continuous hazard monitoring, including relating the internal magma migration to the continuous seismic observations. Using machine learning strategies, we will enhance the detection and the characterization of the seismicity observed at Mount Fuji to model the relationship between the statistically inferred classes of seismic signals and the potential types of volcanic activities. We will conduct comparative experiments with more active volcanoes such as Mount Asama. The project will evaluate seismic signals recorded by classical seismometers and fiber optic strain-meters, providing a new objective insight into volcano-seismology.
The first short-term objective of the PhD is to inspect and prepare the seismic data for large-scale calculations such as tomography and classification (second and third objectives). The seismic data from Mount Fuji is available from 18 permanent seismic stations over more than two decades (from 2000 to 2022) and comes with a local seismicity catalog detected by the Japan Meteorological Agency (JMA). We will also select a dataset of moderate teleseismic events for local waveform tomography at up to 2 Hz to construct a background model for back propagation. We will build a similar dataset for Mount Asama over the same period of time from 20 permanent stations. We will conduct the local waveform inversion with the Direct Solution Method Kernel Suite (DSM Kernel Suite, Fuji et al. 2012) to facilitate local waveform inversion for structures beneath the seismic array with the aid of spectral element method Specfem3D (Monteiller et al. 2013). We will discuss an efficient way of meshing volcanoes and modify the code to compute strains along lines for further analysis of fiber optic experiments in Mt Asama and Mt Stromboli. Even at the target resolution of a few kilometers, we will not be able to detail the magma chambers within the volcano body. But this model should be efficient enough to pursue back projection of seismic sources using the full resolution matrix. We will check the validity of the obtained model(s) by investigating several forward modeling experiments. We will perform the same procedure for Mt Asama as well for the further comparative studies.
The main difficulty of detecting interpreting local seismic sources in volcanoes is its complex feature that cannot be well explained with a point source (Maeda & Takeo 2011). The low-frequency signals of these local seismic events could infer magma migration, which can be expressed as an extended time-lapse volumetric (4D) source mechanism. Satriano et al. (2014) showed the efficiency of the back projection method proposed by Kawakatsu & Montagner (2008), which is mathematically equivalent to the cross-correlation of data acquired with an array of data and Green’s functions. In the framework of one-station InSight project on Mars, we showed that this method can further work if we take into account the inverse of the resolution matrix
(correlations of Green’s functions) even for one-station data (Jacob et al. 2022). In this task, we will first store the Green’s functions, computed for the model obtained in the first part of the PhD for 18 permanent stations and prepare the inverse of the resolution matrix (size of the number of points in 3D solid volcano squared) by iterative methods. This will produce a catalog of movies that localize 4D seismic sources for ~100 events per year for Mount Fuji and between 100 and 300 events per year for Mt Asama. Since Mt Asama is active and has long-lasting observation records, we will be able to compare the 4D images to the eruption history. We will then make use of the waveforms and the 4D images to relate the observed seismic signals with volcanic activities, with AI-based regression. Following Falcin et al. (2021), Seydoux et al. (2022) and Journeau et al. (2022), we will build a deep neural network to solve the regression problem, and identify the (potentially nonlinear) relationship between observed seismicity and the 4D images, revealing potentially useful signatures for volcanic activity forecasting. Since dealing with waveforms is a complex task, we will investigate various transformations that can help solve the task while keeping the algorithm complexity as low as possible to ensure stability and operability. If successful on the several volcanoes we identified, we also plan to conduct direct comparisons of the learned representation between the volcanoes.
This project will be conducted in collaboration with ERI to bridge methods, data and instrumentation. In addition to the supervisors, we will closely interact with Nobuaki Fuji (IPGP), Yosuke Aoki from (ERI), Stéphanie Durand (CNRS, ENS Lyon), Claudio Satriano (CNAP, IPGP), Akiko Takeo (ERI) and Éléonore Stutzmann (CNAP, IPGP).